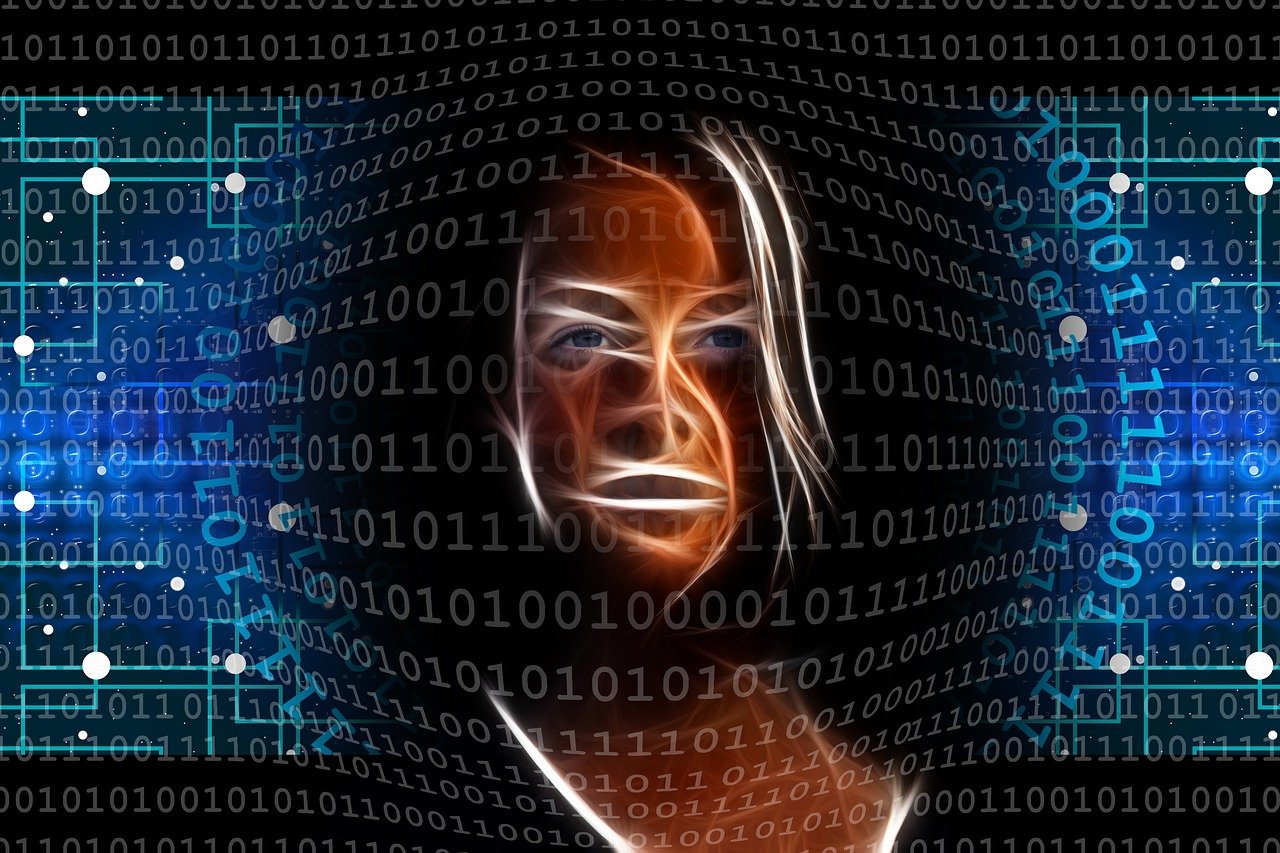
Beyond Siri: The Future of Natural Language Processing in AI
Artificial intelligence (AI) has been making strides in recent years, particularly in the area of natural language processing (NLP). NLP is the subfield of AI that deals with the interaction between computers and human language, and it enables computers to understand, interpret, and generate human language. With the advent of NLP, machines can now perform tasks such as language translation, sentiment analysis, and speech recognition with increasing accuracy. This article will explore the current state of NLP and its future prospects.
What is the current state of NLP?
Natural Language Processing (NLP) is a rapidly evolving field of Artificial Intelligence that has seen significant progress in recent years. With advancements in machine learning and deep learning, NLP algorithms have become more sophisticated, allowing machines to better understand human language and produce human-like responses. One of the most well-known examples of NLP is Siri, the voice-activated personal assistant on Apple devices. Siri is able to recognize voice commands and respond with the appropriate information or action. However, Siri’s capabilities are limited to a narrow range of tasks, such as setting reminders, sending messages, and answering general knowledge questions.
Another major breakthrough in NLP is the development of pre-trained language models like BERT (Bidirectional Encoder Representations from Transformers) and GPT (Generative Pre-trained Transformer). These models have significantly improved the accuracy of language models, making them capable of tasks such as text classification, sentiment analysis, and machine translation.
In addition to these known examples of NLP, we have also seen the rise of conversational AI chatbots, which have become increasingly popular in industries such as customer service and healthcare. These chatbots can understand natural language queries and provide responses in real-time, improving the user experience and reducing response time.
Despite these advancements, there are still significant challenges to overcome in NLP. One major challenge is the lack of diversity in training data, which can lead to biases in NLP algorithms. For example, if an NLP model is trained on data that is biased against a certain group of people, it may produce biased responses when interacting with that group.
There is also the difficulty of understanding contextual meaning in language. Natural language is inherently ambiguous and context-dependent. The same word or phrase can have multiple meanings, depending on the context in which it is used. Additionally, language is constantly evolving and changing, which makes it difficult for machines to keep up. While NLP algorithms have become better at understanding individual words and phrases, they struggle with understanding the meaning of words in context. This can lead to misinterpretations and errors in the responses they generate.
Though these challenges are significant, the future of NLP still looks promising. Researchers are continually working to improve NLP algorithms by developing more sophisticated models and training them on diverse datasets. As NLP technology continues to improve, we can expect to see it increasingly integrated into various industries and applications, including intelligent virtual assistants, personalized advertising, and predictive analytics.
Future Prospects of NLP
One of the most significant areas of future development in NLP is in the field of conversational AI. As NLP algorithms become more sophisticated, they will be able to engage in more natural and human-like conversations with users. This will have implications for a range of industries, from customer service to healthcare, where conversational AI could be used to improve patient interactions and outcomes.
Another area of future development in NLP is in the realm of machine translation. While machine translation has come a long way in recent years, there is still much room for improvement. As NLP algorithms become better at understanding the nuances of language, we can expect to see more accurate and nuanced translations between languages.
NLP also has significant potential in the field of sentiment analysis, where it can be used to analyze large volumes of text data to understand how people feel about a particular topic. This could be used in a range of contexts, from social media monitoring to market research. Another area of interest is the development of more advanced language models, such as OpenAI’s GPT-3. GPT-3 is a language model that is capable of generating human-like text, such as articles and essays, based on a given prompt. This technology could have significant implications for the field of content creation, as it could automate the process of generating written content.
One other exciting area of development in NLP is in the field of personalized AI assistants. As NLP algorithms become more advanced, they will be able to understand and respond to individual users’ needs and preferences more accurately. This could have significant implications for industries such as healthcare, where personalized AI assistants could be used to help patients manage chronic conditions and improve their overall health.
While the future of NLP is looking promising, there are also challenges that must be addressed to ensure that this future is both beneficial and ethical. One of the most significant challenges is bias in NLP algorithms. As we have seen with other AI applications, bias can be inadvertently built into algorithms, resulting in discriminatory outcomes. It is essential to ensure that NLP algorithms are developed in a way that is free from bias and that they are regularly audited to identify and address any issues that arise.
Another challenge is the ethical use of NLP data. As NLP algorithms become more advanced, they will be able to process large volumes of sensitive data, such as health records and personal communications. It is essential to ensure that this data is used ethically and in compliance with relevant data protection regulations.
Despite these challenges, NLP is poised to make significant strides in the coming years. One area of particular interest is the development of more sophisticated chatbots. Advances in machine learning and deep learning algorithms are allowing chatbots to become more conversational and interactive, which could make them more useful in a variety of applications, such as customer service and healthcare.
NLP is an exciting area of AI research that has the potential to revolutionize the way we interact with computers. While there are still challenges to overcome, such as the issue of bias and privacy concerns, the future prospects of NLP are promising. As AI technologies continue to evolve and improve, we can expect to see more sophisticated and intelligent natural language processing tools emerge.
References:
- Liu, X., & Zhang, Y. (2017). Recent developments in deep learning for natural language processing. Journal of Information Science, 43(3), 305-317.
- Kelleher, J. D., & Tierney, B. (2018). Data science and machine learning: challenges and opportunities for the systems and control community. Annual Reviews in Control, 46, 250-259.
- OpenAI. (2020). GPT-3. https://openai.com/gpt-3/
- Devlin, J., Chang, M. W., Lee, K., & Toutanova, K. (2018). BERT: Pre-training of deep bidirectional transformers for language understanding. arXiv preprint arXiv:1810.04805.