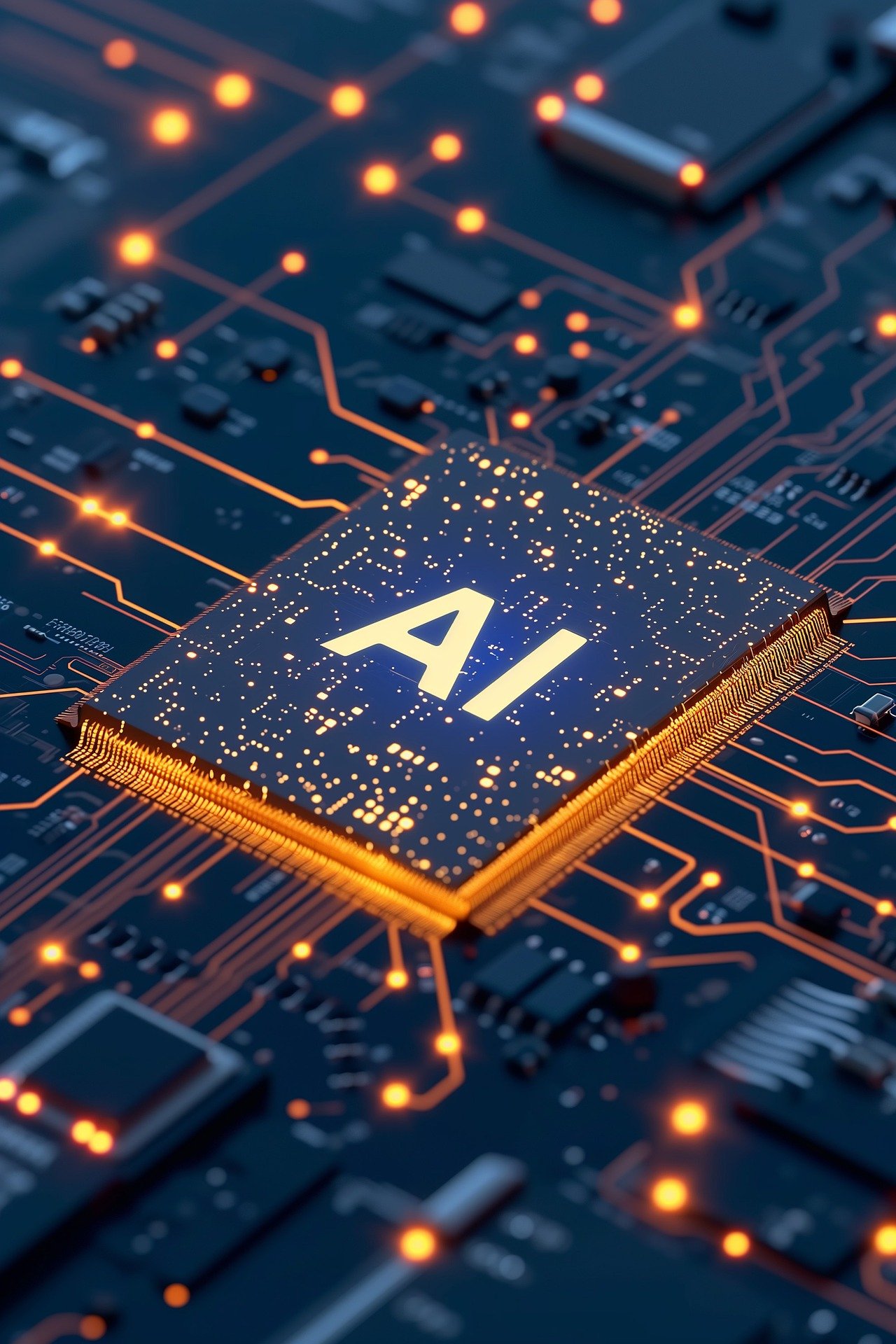
Exploring Explainable AI: Bridging the Gap Between AI Decision-Making and Human Understanding
Artificial Intelligence (AI) systems have increasingly become integral parts of our lives, shaping decisions in areas such as healthcare, finance, and criminal justice. However, as AI algorithms become more complex and pervasive, there arises a critical need for transparency and accountability in their decision-making processes. This is where Explainable AI (XAI) comes into play, offering a bridge between the opaque nature of AI algorithms and human comprehension. In this article, we delve into the concept of Explainable AI, its significance, applications, and the ongoing efforts to enhance transparency in AI systems.
Understanding Explainable AI
Explainable AI refers to the set of techniques and methodologies aimed at enabling humans to understand the rationale behind AI algorithms’ decisions. Unlike traditional “black-box” AI models, which generate outcomes without providing insights into the underlying reasoning, XAI techniques strive to provide interpretable explanations for AI-driven decisions. By shedding light on the decision-making process, XAI enhances trust, accountability, and fairness in AI systems, thereby facilitating their acceptance and adoption across various domains.
Significance of Explainable AI
The importance of Explainable AI cannot be overstated, particularly in high-stakes applications where AI decisions have profound implications for individuals and society. In healthcare, for instance, XAI can help clinicians understand the factors influencing diagnostic recommendations or treatment plans, enabling them to validate AI-driven decisions and identify potential biases or errors. Similarly, in finance, Explainable AI can provide investors with insights into the rationale behind algorithmic trading strategies, enhancing transparency and mitigating risks.
Applications of Explainable AI
Explainable AI finds applications across diverse domains, ranging from healthcare and finance to criminal justice and autonomous systems. In healthcare, XAI techniques are leveraged to interpret medical imaging results, predict patient outcomes, and recommend personalized treatment plans, empowering clinicians to make informed decisions based on AI-generated insights. In finance, XAI algorithms are employed for credit scoring, fraud detection, and risk assessment, allowing financial institutions to explain the factors contributing to loan approvals or transaction verifications.
Challenges and Future Directions
Despite its promises, Explainable AI poses several challenges, including the trade-off between model complexity and interpretability, the need for scalable and efficient XAI techniques, and the inherent tension between transparency and performance. Addressing these challenges requires interdisciplinary collaborations between AI researchers, ethicists, policymakers, and domain experts to develop robust XAI frameworks that prioritize both accuracy and interpretability. Additionally, ongoing research efforts focus on advancing XAI techniques such as feature attribution methods, model-agnostic explanations, and interactive visualization tools to enhance human-AI collaboration and decision-making.
Explainable AI represents a pivotal advancement in the quest for transparent, accountable, and trustworthy AI systems. By enabling humans to comprehend AI-driven decisions, XAI not only enhances trust and acceptance but also empowers individuals to validate, scrutinize, and ultimately, harness the potential of AI technologies for societal good. As we navigate the increasingly complex landscape of AI, the pursuit of Explainable AI serves as a guiding principle towards realizing the full benefits of artificial intelligence while mitigating its risks and challenges.